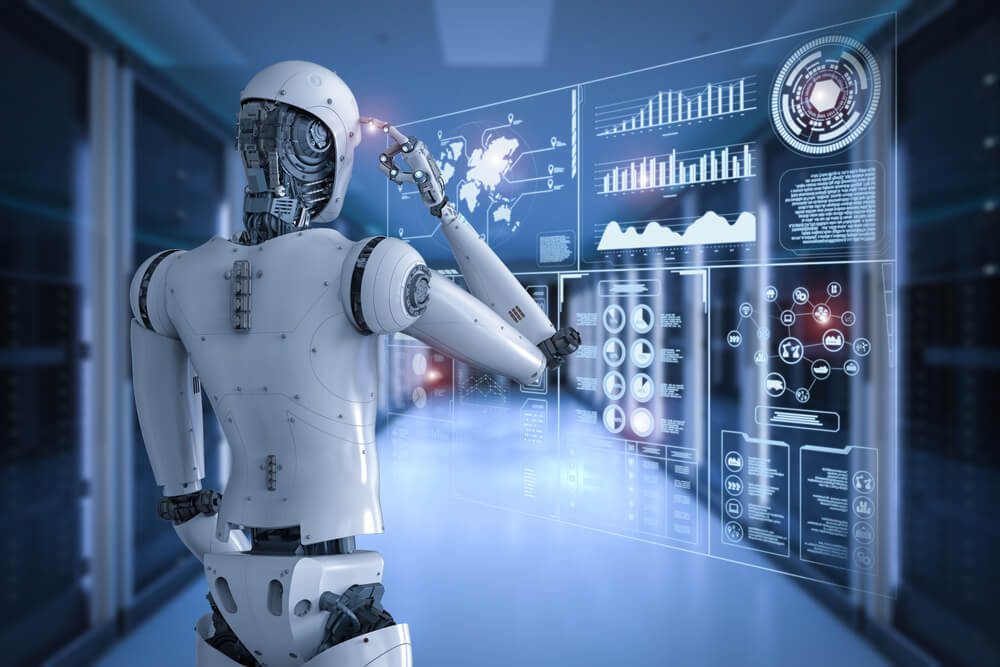
The Future of AI and Digital Health
In a sense, Artificial Intelligence and digital health were made for each other. Digital health looks for digital solutions to promote the health of the body and the mind; AI attempts to reproduce feats of the mind in digital form. Digital health is the matter; AI the mind.
In fact, AI is already making a huge impact on the digital health sector … and that’s just the opening salvo. Consumers, medical providers, government regulators, and funders of startup companies are all coming into alignment. The union of AI and digital health stands to completely upend everything about how we practice healthcare, from how we submit blood tests, to how we monitor chronic conditions, to our very relationships with our doctors.
No time to read this article? Watch the video instead.
What Is Artificial Intelligence?
Artificial intelligence is poorly understood. It is sometimes defined as “the ability of a machine or computer to perform tasks that usually require human intelligence.” But that definition is incomplete. After all, historically it took a human brain to add 2 + 2, but electronic calculators have been with us since the ‘60s. So what is different about AI?
Let’s back up and take a moment to appreciate what we really mean when we say human intelligence. The textbook definition of “human intelligence” has two parts …
- The ability to learn, understand or deal with new and/or challenging situations.
- The ability to apply knowledge to manipulate one’s environment or to think abstractly as measured by objective criteria.
So to mimic a human mind, AI must be able to learn and apply knowledge to affect its environment. One of the distinguishing characteristics of AI is that, when applied to a specific task, it tends to perform that task better than any one specific human mind. For example, Google AI is able to detect breast cancer more accurately than some human doctors.
AI, therefore, is not just a machine that is able to perform tasks that normally require human intelligence. To be classified as AI, the software must be able to solve a problem or produce an outcome better than any single human can.
This definition means different things to different people or different tasks. AI technicians generally recognize four stages of AI, only two of which are technologically feasible right now.
1. Reactive Machines AI
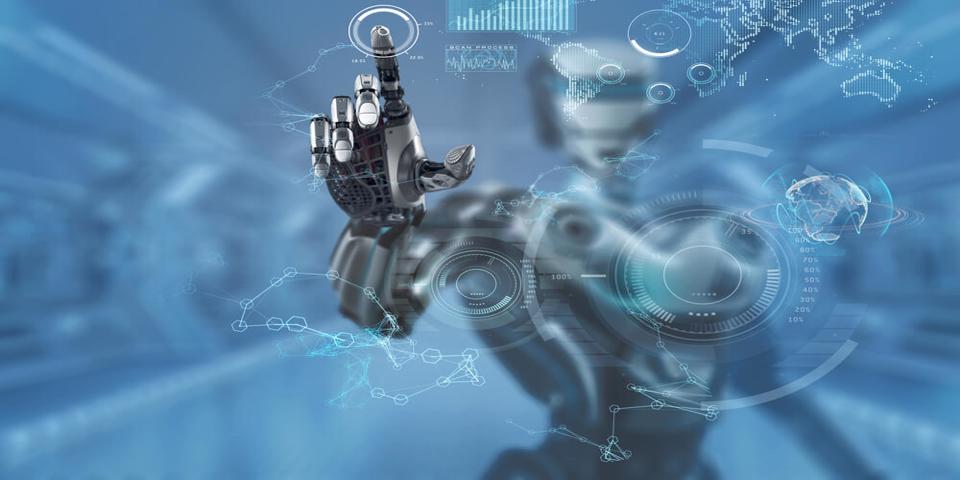
In the 1980s, IBM invented the Deep Blue super chess playing software, which was able to beat champion chess players. The tech world was stunned. What was going on? A computer could beat our smartest chess players. Had computers become smart?
Not exactly. This earliest conception of AI falls into the category of “reactive machines.” Deep Blue was programmed to read the conditions of the board and use predictive data to determine the next move that, by extrapolative data, was most likely to eventually result in a checkmate.
Yes, it meets our definition of AI. By beating Gary Kasparov, the world chess champion, in a six-game rematch, Deep Blue exhibited the ability to perform a task or solve a problem better than any one human mind could.
However, reactive machines do not learn. Deep Blue benefitted from the fact that there are strict rules to chess, with only so many inputs and outcomes. Once they were all programmed, Deep Blue could reliably make the highest-potential chess move, every time. But that’s all it could do—read the situation and respond according to its programming. Google’s AlphaGo, which routinely beats talented Go players, is another example of a reactive machine.
Reactive machines are the most primitive type of AI that fits our definition, and AI technology had a long way to go before breakthrough medtech was on the horizon.
2. Limited Memory AI
Today’s AI technology resides here—Step 2 on a hypothetical AI ladder. Limited memory AI outdoes reactive machines by being able to look into the past in a limited way.
For example, self-driving cars cannot judge their surroundings and make navigational or safety decisions based on a snapshot in time, the way Deep Blue could with the snapshot of the state of a chess board. Self-driving cars must judge proximity and collision likelihood with moving and stationary objects around it, determining where objects are likely to be in the future based on where they are now and where they were in the past.
This assessment of vectors mimics the decision-making of a human driver. In fact, like Deep Blue always knowing the best chess move, self-driving cars may be able to make better reactive decisions than a human driver, although we’re still working out how to make self-driving cars sensitive to the needs of human life. For example, how do we stop a self-driving car from swerving to avoid a collision and hitting a pedestrian, reading the pedestrian as the lesser “threat?”
But even with its limited memory, we start to see AI having an impact on medical technology in the form of:
- Cancer Diagnostics. Doctors use limited memory AI machines to more accurately and efficiently diagnose cancer from an MRI. They do this by feeding hundreds of example MRIs that exhibit the cancer into the machine’s memory, where it can tease out details and markers that humans miss. Once calibrated (“taught”), the machine can spot cancer on an MRI more effectively than humans.
- Robot Dentists. Robotic arms can be programmed to perform procedures like the insertion of dental implants, using haptic feedback to detect particularities of the patient. Chinese technicians developed the first robotic dentist that performed a successful dental implant procedure without human involvement.
- Virtual Nurse Assistants. Using similar technology as AI chatbots or voice-activated assistants, virtual nursing assistants like BU’s iCare Navigator can offer advice and encouragement, gradually building a “personalized” relationship with the patient.
3. Theory of Mind
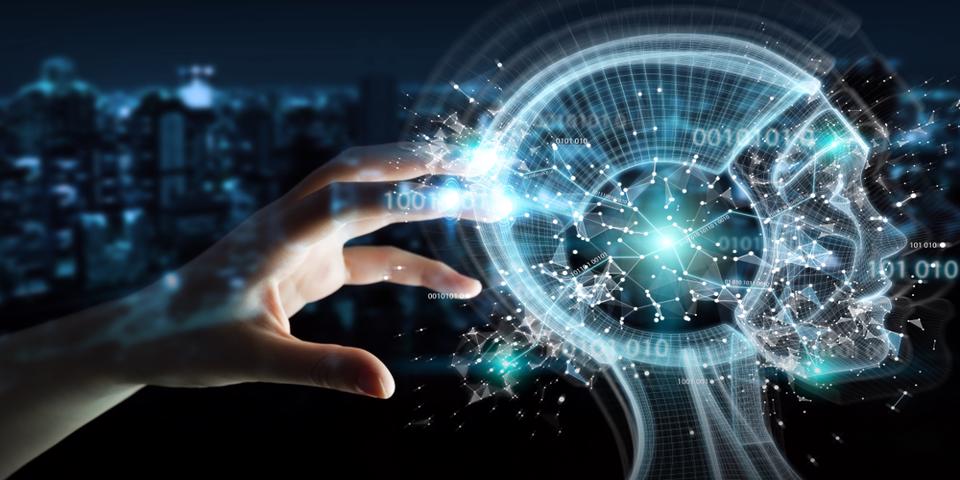
Step 3 of the theorized AI ladder is called “Theory of Mind.” Machines in this category can understand the needs and expectations of humans or other machines, creating a truly interactive machine relationship.
At this stage, we enter the realm of the theoretical. While no working Theory of Mind machines exist, top tech innovators have Theory of Mind in their sights. The closest examples include:
- Google DeepMind. Acquired by Google in 2014, UK-based DeepMind is an artificial neural network designed to learn how to play video games and compete with human players.
- Sophia. This Hong Kong-created humanoid robot is the most prominent attempt to create an AI person. Debuted at SXSW in 2016, Sophia “gave” several interviews before making headlines by being granted Saudi citizenship, making her the first robot to be granted citizenship in any nation.
- OpenAI Five. OpenAI Five is an Elon Musk-owned neural net designed to learn and play the video game Dota 2.
All of these technologies move in the direction of Theory of Mind, but none of them are perfect examples of the technology.
4. Self-Aware AI
At the top of the food chain of AI is Level 4—self-aware AI. This level is characterized by autonomous machines that understand their own existence and can make decisions on their own.
As you might have guessed, no tech we have today even comes close to self-aware AI. This is strictly the province of Star Trek: Picard, Westworld, and Terminator movies.
Examples of AI Currently at Work in Healthcare
While we may be stuck at Stage 2 on the AI rubric, the good news is that there’s a great deal of potential in limited memory—an enormous amount of work is being done in this field, and there’s a lot still to be done. Examples of AI currently at work in healthcare include:
Machine Learning
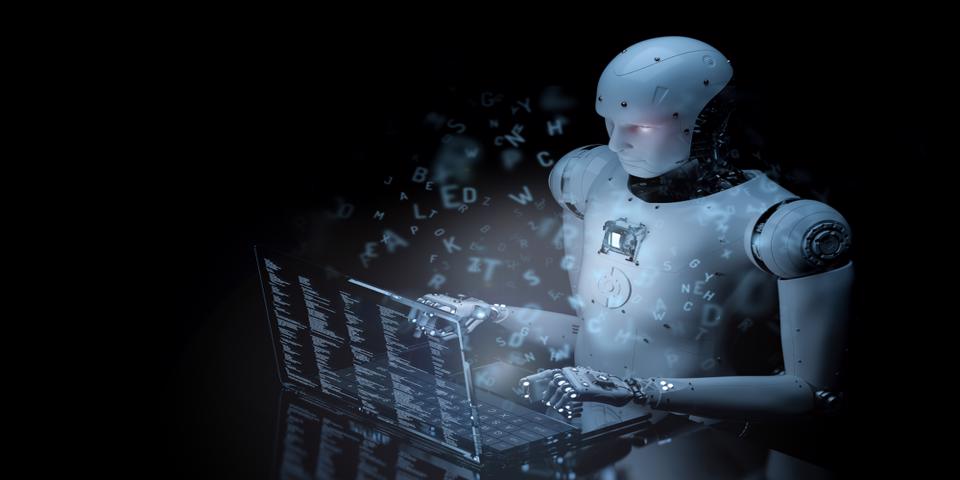
Computers capable of machine learning (ML) examine algorithms and improve their ability to perform tasks through experience, similar to the way humans learn from experience. The algorithms a computer examines are called “training data,” and a computer uses the data to build a mathematical model for future decision-making.
This might sound sophisticated—and it is—but we also interact with it every day. Your email client’s spam filter is capable of machine learning. Over time, the spam filter comes to identify spam more efficiently, based on your message-opening habits.
Examples of machine learning at work in medtech include the Kinsa Smart Thermometer. This consumer medical device not only takes fast and accurate temperature details, but it also tracks temperature records for each user and uploads them to a cloud-based app. The thermometer can suggest possible diagnoses, recommend treatments, and even allow users to create a “local group” function, a kind of social network that informs families what diseases might be going around in their social circles or those of their children.
This allows the Kinsa Smart Thermometer to do much more than detect symptoms of disease—it can warn of disease and possibly even help families prevent disease.
Natural Language Processing
Amazon Alexa, Apple’s Siri, and the Google Home Assistant all function thanks to natural language processing (NLP), a subset of AI technology that melds linguistic and computer science to create machines you can talk to and that can respond.
Alexa can already learn healthcare skills, while virtual note-taking and virtual nursing assistants continue to expand the footprint of NLP in medicine.
Robotics
Many peoples’ conception of robots is still perilously colored by Star Wars. Robotics isn’t about creating artificial humans so much as it’s about addressing the ability of computing and engineering science to create mechanical assistants for human beings.
Robots are programmed to perform repetitive assembly tasks on factory floors or repetitive consumer tasks like vacuuming the floor. Robots have the advantage of never getting tired and not being susceptible to human error (though they are susceptible to other kinds of errors).
Examples of robots in medtech include:
- Robotic Dentists. Mentioned before, robotic arms programmed with haptic feedback have already successfully inserted dental implants without the aid of human input.
- The Monarch Platform. This robotic endoscope helps doctors access the lungs with minimally-invasive procedures, controlling the endoscope with a videogame-like controller to aid in the collection of biopsies and identification of cancers deep within the lungs.
- Robotic Surgical Systems. Among pioneering surgical systems is the Da Vinci System, an assembly of robotic arms that hold cameras and surgical tools, which allows doctors to perform heart surgery and other surgeries from afar.
Machine Vision
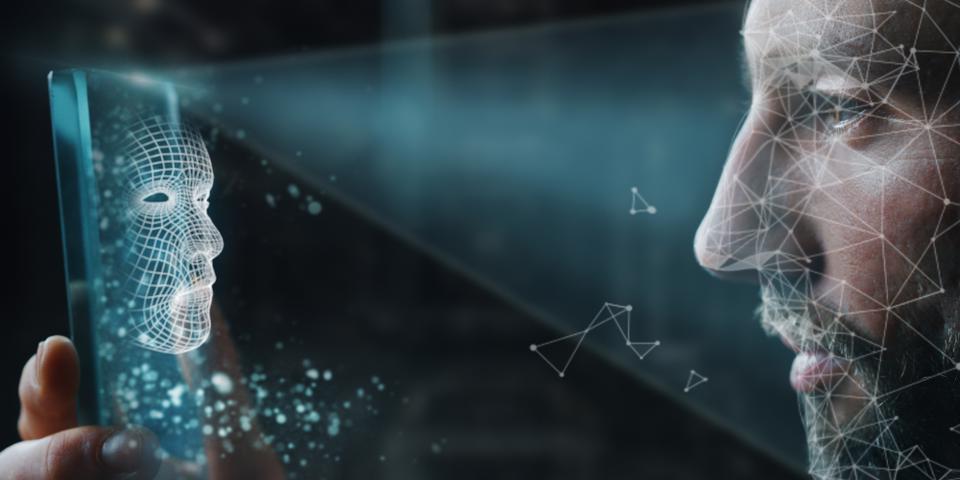
Machine vision (MV) is the engine that drives Google Reverse Image search. Rather than having to search for an image, a user is able to input an image and find its source. Google’s search algorithm also uses MV instead of alt text to identify relevant images for the purposes of SEO and image searches.
MV is not limited to search, either. Various industries use MV to guide robots, inspect inventory, and control processes. It is simply a process by which a machine receives visual imagery as input and performs analysis on it. This might seem simple, but this is a task that usually requires eyes. Earlier computing technology was only able to analyze bits of data (1s and 0s).
Swoop Aero Drones offered a dramatic example of MV in medtech. These drones were used to deploy vaccines and medications to barely-accessible locations in the Democratic Republic of Congo, one of the poorest countries in the world. Deployed by the UN, USAid, and UKAid, these drones used MV to identify their delivery targets.
What Is Digital Health?
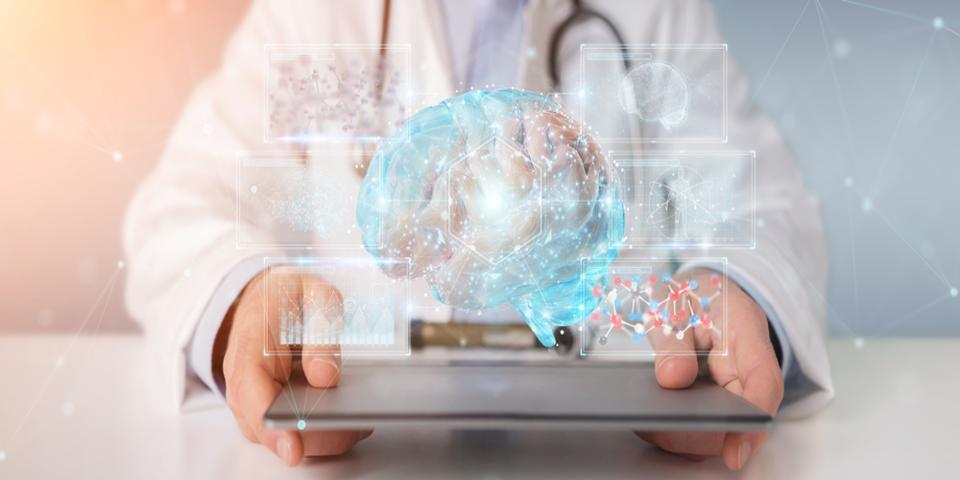
Steve Jobs predicted the impact of digital health when he said, “I think the biggest innovations of the 21st Century will be at the intersection of biology and technology.”
The digital revolution has touched nearly every aspect of our lives, but digital health is only just getting started. Digital health allows medical professionals and patients to work together toward managing health conditions via revolutionary digital tools.
The Healthcare Information Management Systems Society (HIMSS) offers the following definition of digital health:
“Digital health connects and empowers people and populations to manage health and wellness, augmented by accessible and supportive provider teams working within flexible, integrated, interoperable, and digitally-enabled care environments that strategically leverage digital tools, technologies, and services to transform care delivery.”
Under this broad definition, digital health takes many forms, including:
- Telehealth. The use of teleconferencing and eCommerce to provide patients with access to medical services they previously accessed only at a hospital or clinic, like consultations, diagnoses, prescriptions, and prescription fulfillment.
- Wearable tech. Devices like the FitBit, Apple Watch, and implanted devices can measure heart rate, blood glucose, sleep patterns, and other health indicators to provide medical professionals with data not available at a clinical visit.
- Big Data. Medical device and software technology able to collect and process data, facilitating better diagnoses, better treatment plans, and ultimately producing better outcomes.
- Imaging and Diagnostics. Tech solutions to produce better medical imaging and better diagnostics.
- Smart Insurance and Pricing. Intelligent platforms to price insurance and drugs.
- Genomics. The use of powerful computers to map and analyze genomes to identify risk factors, abnormalities, and indicated treatments.
- Providers and Concierge Technology. Streamlined staff and patient intake, resulting in less waiting room time and fewer clipboards to fill out.
Venture capitalists have taken notice. VC funding of digital health ventures ballooned from $211 million in 2010 to a peak of $9.4 billion in 2018, before barely retreating to $8.8 billion in 2019. Three healthcare startups were valued at over $1 billion, including:
- Grail (cancer detection), funded at $1.78 billion.
- Oscar (insurance), funded at $1.3 billion.
- Bright Health (insurance), funded at $1.1 billion.
[bctt tweet="VC funding of digital health ventures ballooned from $211 million in 2010 to a peak of $9.4 billion in 2018, before barely retreating to $8.8 billion in 2019." via="no"]
Understanding the Rise of Digital Health
Why the sudden explosion in valuation and VC interest in digital health?
A big driver of the trend is the explosion in popularity of smartphones, a digital device that consumers personalize and have on them at all times. Smartphone ownership in the US has ballooned from 62.6 million smartphones in 2010 to 236.8 million smartphones in 2020, almost quadrupling and accounting for a smartphone in the pockets or purses of almost two-thirds of all Americans.
Steve Jobs’ brainchild company, Apple, purveyors of the world’s most popular smartphone, led the way in underscoring Jobs’ prediction that tech and biology would intersect. In 2014, Apple launched HealthKit, a software development kit that app developers could use to design apps specifically for iOS (iPhone, iPad) and WatchOS (Apple Watch).
Developers responded with vigor. The first apps integrated with HealthKit launched in 2017. By 2020, the App Store was stocked with over 320,000 health apps. Apple followed up HealthKit with Research Kit, a platform that allows medical researchers to adapt the iPhone as a data-collection tool for their studies.
[bctt tweet="Today, the Apple App Store hash over 320,000 health apps." via="no"]
Having already broken ground on wearables, data storage, and digital health app development, Apple continues to lead the way in digital medicine disciplines, including AI-friendly sectors like telemedicine, coaching, and healthcare AI chatbots.
In a way, smartphones are the first “wearable” device, and as we will see they have the potential to spare as medical devices in their own right. Further, a data-capable device in everyones’ pocket expanded the notion of what was possible in a digital world. You can search for facts or buy products on a smartphone; why not use the smartphone as a hub for something as personal and critical as managing your health, wellbeing, and longevity?
Consumers and VCs aren’t the only drivers of this trend toward digital health. The Food and Drug Administration (FDA), the US federal regulatory body tasked with approving medications, medical devices, and other medtech, has demonstrated an increasing taste for digital health.
The FDA has approved over 50 digital health solutions in the last six years, most of them falling into one of three categories:
- Cancer detection (breast, liver, lung).
- Treatment of chronic conditions (ADHD, diabetes).
- Prediction of diseases (atrial fibrillation, sleep disorders, stroke).
In addition to the FDA, doctors are getting into the act. 90% of doctors surveyed see the advantage of digital health tools in the fulfillment of their mission to prolong and improve lives. The survey found that doctors are already using digital health tools in the following ways:
- Providing consumers with access to clinical data. (58%)
- Point-of-care workflow enhancements. (47%)
- Clinical decision support. (37%)
- Patient engagement. (33%)
- Telemedicine. (30%)
- Remote monitoring and management for improved care. (22%)
- Remote monitoring for efficiency. (16%)
In short, digital health stands to benefit everyone in the chain of medical service, including:
- Patients, by offering increased access to expert analysis, greater visibility and control over health information, personalized medication, greater privacy for sensitive medical information, and an overall superior patient experience.
- Providers, enabling them to offer more effective and targeted treatment, comply more easily with HIPAA, provide superior care quality and efficiency, novel treatment options for rare diseases, and shift the focus from treatment of disease to prevention of disease.
- Payers, reducing the complexity of the healthcare system, cutting costs by improving care and clinical outcomes, exposing greater patient populations to effective treatments, offering superior support experiences, and decreasing the economic burden on payers.
Ways AI Has Pushed Digital Health Forward
Advances in AI have been some of the most meaningful breakthroughs in technology in decades. Digital health has benefited from these innovations in numerous ways, including:
mHealth (mobile health)
The World Health Organization defines mHealth as “the medical and public health practice supported by mobile devices, such as [cell] phones, patient monitoring devices, personal digital assistants (PDAs), and other wireless devices.”
We’ve already talked about the explosion in popularity of mobile devices in the last ten years. mHealth merges that trend with the popularity of digital health, deputizing the smartphone in most of our pockets for medical purposes like:
- Education and awareness.
- Diagnostic treatment and support.
- Disease and epidemic outbreak tracking.
- Healthcare supply chain management.
- Remote data collection.
- Remote monitoring.
- Healthcare worker telecommunication and training.
- Telehealth/telemedicine.
- Chronic disease management.
Smartphones and other mobile devices have obvious advantages in delivering these benefits—they can access the internet, make calls, run apps, take photos and videos, etc. But lesser-known features of smartphones open up even more uses.
A smartphone’s in-built microphone enables it to record breathing patterns to aid in cardiopulmonary monitoring. Many smartphones also have a built-in triaxial accelerometer, which detects whether the phone is level or upright. This motion-sensitive device makes the mobile device useful in the diagnosis and monitoring of motor disorders.
Between 2014 and 2018, the National Institute of Health (NIH) offered 397 grants to mHealth apps, totaling $138.1 million dollars in grant money, directed to the following categories:
- Monitoring and Feedback (192 grants).
- Skills Training (85 grants).
- Education and Information (85 grants).
- Cognitive and Behavioral Therapies (68 grants).
- Facilitating, Reminding, and Referring (60 grants).
AI disciplines at work in mHealth include:
- Machine Learning. The data collected by mHealth devices act as training data for limited memory machines used to detect, diagnose, and track diseases, as well as create reminders that improve treatment-plan adherence.
- Natural Language Processing. Mobile-enabled NLP apps allow users to ask questions and get answers for the purpose of education, information, and skills training.
- Machine Vision. Imagery from mobile devices can be analyzed for the purpose of diagnosis and disease tracking.
[bctt tweet="Between 2014 and 2018, the National Institute of Health (NIH) offered 397 grants to mHealth apps, totaling $138.1 million dollars in grant money" via="no"]
At-Home Testing
Is there any more accepted truism than the notion that you have to go into the hospital or clinic to obtain key diagnoses? Especially diagnoses that depend on lab analysis of bodily fluids like blood, urine, stool, mucus, or saliva?
That truism is becoming outdated, as digital health service providers innovative methods of delivery for testing kits that bring lab analysis in line with the dominance of eCommerce. You can get teeth-whitening kits and pregnancy tests delivered, so why not home testing kits for various diseases?
The answer used to be, because sometimes the samples require more sophisticated analysis to make an accurate diagnosis. AI developers grasped that there needs to be a way not only to collect the sample, but also to return the sample safely, and then provide the consumer with accurate test results in a timely fashion.
The challenges have been significant, but the offerings are getting better and better. From sample collection kits to determine your genomic ancestry or to register as a bone marrow donor, consumers can now order home testing kits for:
- HIV (Oraquick, $40, 92% accuracy).
- Strep (Rapid Response, $40, 97% accuracy).
- Allergies (MyAllergyTest, $53, 97% accuracy).
- Urinary Tract Infection (AZO, $10, 95% accuracy).
- Thyroid TSH Test (Home Health Testing, $31, 95% accuracy).
- Celiac Disease (imaware, $99).
These quick, convenient, cost-effective, and confidential tests are expected to catch on in a big way. The global home-testing market is expected to grow 3.98% year-over-year, tipping the scales at $6.53 billion by 2025.
Chronic Conditions Management
A subset of mHealth apps worth special mention are those that help patients manage chronic conditions. A defining factor of chronic conditions is that they don’t stop when you leave the doctor’s office. Between doctor visits, the conditions persist, presenting a management burden for the patient and a “fog of war” during which the care provider traditionally had no access to data on the patient’s ongoing condition.
Apps for the management of chronic conditions push back against that understanding, providing patients with tools not only to manage their chronic conditions more effectively but also to supply their care providers and doctors with ongoing data to monitor the effectiveness of (and adherence to) treatment plans and calibrate those treatment plans for the best results.
Apps targeting chronic condition management that have been approved or cleared by the FDA include:
- Alivecor. This app allows patients with cardiovascular conditions to record 6-lead EKG readings from their smartphone and share them with their doctors, allowing the doctor to tailor the treatment plan to the patient profile.
- Welldoc’s BlueStar. Type 1 diabetes is a difficult disease to manage, with potentially dire consequences. The BlueStar app pairs with a Continuous Glucose Monitor (CGM) to help patients with T1D monitor their glucose carbohydrate, and insulin levels, as well as track, their food and easily access information about T1D.
- GoSpiro. GoSpiro is an air-capacity measurement device that pairs with a smartphone app to help cardiopulmonary patients measure lung function by blowing into the BlueTooth-enabled device.
AI plays a key role in the function of apps designed to monitor chronic conditions thanks to:
- Machine Learning. Apps that monitor chronic conditions aid in data collection, which acts as training data to limited-memory machines that track diseases and patient data, recommending the best treatment plan.
SaMD
Digitally-enabled medical devices use software. Software, for example, must be used to guide the magnet in an MRI machine. Separate that software from the MRI machine, however, and the software is pretty useless. This kind of software is often called software in a medical device (SiMD).
Software as a medical device (SaMD) is different. As the name implies, the software is the medical device. Whatever device it is loaded into—smartphone, computer, watch, etc.—the software itself performs the medical function in question.
SaMD is also distinct from:
- Software as an accessory to a medical device.
- General purpose software that is not a medical product in and of itself.
As defined by the International Medical Device Regulators Forum (IMDRF), SaMD is defined as “software intended to be used for one or more medical purposes that perform these purposes without being part of a hardware medical device.”
Examples of SaMD cleared or approved by the FDA include:
- MedOptimizer. The smartphone app monitors, in real-time, symptoms of ADD and ADHD, as well as side effects of medications, to help optimize treatment plans.
- Mole Mapper. This smartphone app lets patients photograph and keeps track of moles in an effort to achieve early detection of potentially dangerous cancers or lesions.
- EpSMon. This smartphone app uses the phone’s accelerometer to track symptoms of epilepsy, keeping track of triggers, and risk factors.
AI plays a key role in SaMD through the use of:
- Machine Learning. Cloud-enabled SaMD apps aid in data collection, which acts as training data to limited-memory machines that track diseases and patient data, recommending the best treatment plan.
- Machine Vision. SaMD apps use MV to track moles and other conditions that benefit from visual data.
What Does the Future Look Like?
AI and the devices it powers have only scratched the surface of their potential. By 2020, 50 billion Internet of Things (IoT) devices were in service, including devices that utilize AI and medical devices. By 2030, that number of IoT devices is expected to swell to 500 billion, effectively connecting us in a kind of omnipresent neural network.
Experts believe that we are progressing towards a condition of constant connectivity—“never offline,” enveloped in sensors collecting our data constantly.
Whatever other implications that might carry, it bodes a bright future for healthcare. The more data we collect, the better we can detect early, diagnose, and track the spread of diseases and health conditions, enabling consumers, their medical providers, and healthcare policy makers to take early action.
Specific playgrounds for AI healthcare tech developers currently include:
AI ‘Smart’ Pills
The FDA has given the go-ahead to etectRx’s ID-CAP system, a wireless-enabled digital pill that patients can ingest and pass through their digestive system, transmitting data about the patient’s gut health and other digestive data. Among other functions, it can track treatment-plan adherence by confirming whether or not a patient has actually taken his or her medication.
Invisible Wearables
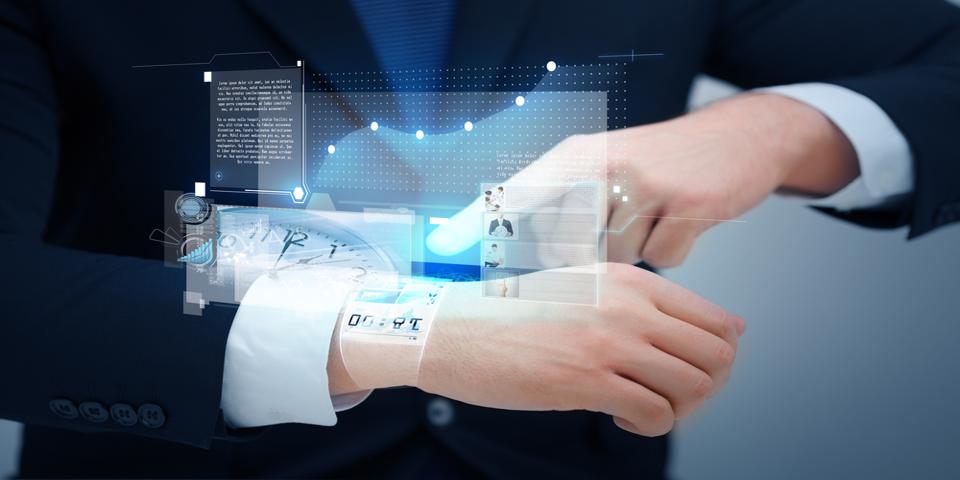
“Wearables” are not limited to smartwatches or heart-rate monitors. Among other brainstorms, South Korean medtech innovators are loading soft contact lenses with sensors to create “smart contact lenses,” which record and transmit data about ocular health to the cloud for use by the patient and his/her doctor.
Fully Immersive Bionic Limbs
In another innovation predicted by Star Wars, scientists are on the verge of prosthetics similar to Luke Skywalker’s bionic hand—a prosthetic limb with neural connectivity, which articulates according to the patient’s thoughts just like a normal limb and transmits sensory data back to the user, again just like a natural limb.
AI Doctors
Much has been made about the potential for AI to render various jobs and professions obsolete. Doctors probably thought they were safe from that fate, and to a certain extent, they are right. Doctors aren’t in danger of being replaced by robots yet, but Androctor is working on it, having produced Anna. AI Dr. Anna has a long way to go, but so far she can interact with patients, answering questions using NLP, observing patients with MV, and calling upon a vast database of knowledge to answer questions.
Conclusion
As we take stock of how far AI has come, and how it has driven advances in digital health technology, it’s easy to be excited by the future. Many questions still remain—how to preserve the security and privacy of medical data, for example, or the unexpected hazards of constant biomedical surveillance.
But with the prevalence of smartphones, wearable devices, AI assistants, and autonomous robots, all of them brimming with medical applications, the future of digital health looks bright. AI integrations have the potential to detect diseases earlier, track epidemics more effectively, laser-target treatment options, and connect patients to their doctors in ways that a pre-smartphone generation never thought possible.
Want To Meet Our Expert Team?
Book a meeting directly here
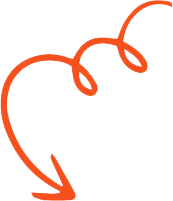