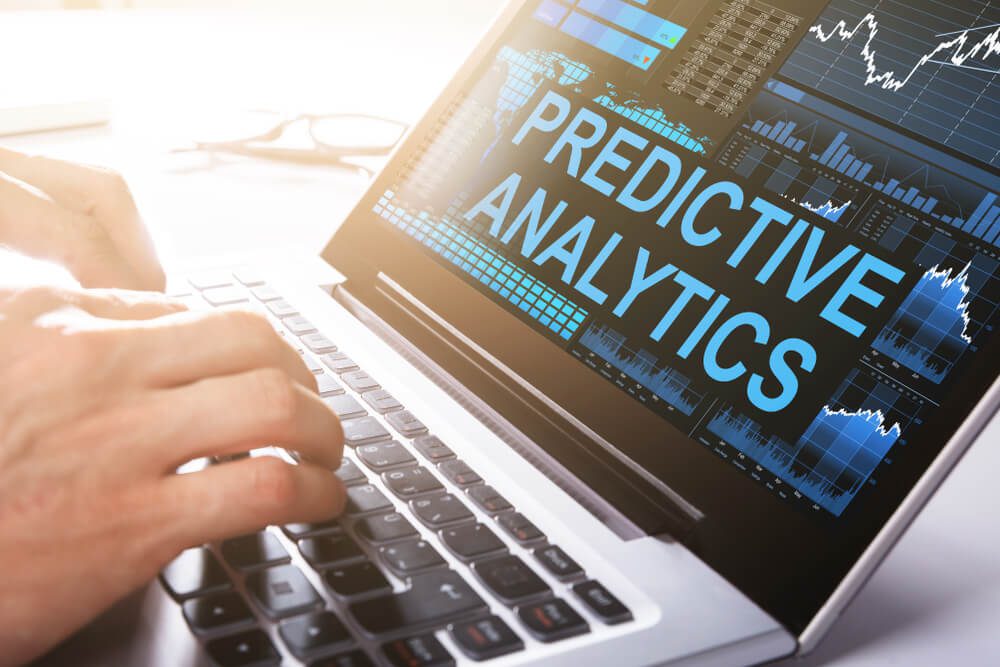
Easy Steps Boost Business With Targeted Predictive Analytics
What do Walmart, Netflix, and Volvo have in common? All have used artificial intelligence (AI) to forecast user needs and develop more effective solutions that increase revenues and customer retention.
If you are a growing Illinois business, you can use the same technology to your advantage with an analytics company in Chicago. This article discusses the essential easy steps in doing predictive analytics:
- Know what you want to predict.
- Collect the data from various sources.
- Clean and prepare the data.
- Pick the best predictive model.
- Train, evaluate, and deploy the model.
What happens at every step? Read this article to learn the basics. Let’s go!
1. Know What You Want to Predict
Predictive analytics helps you forecast outcomes so that you can develop an agile digital marketing plan. However, data is only useful if you know what to do with it.
The table below shows the most common reasons businesses use this advanced analytics technique and their use cases:
Objective | Example |
---|---|
Increase revenue | Chicago-based retailers, especially those on the Magnificent Mile, understand local shopping trends and optimize their inventory to cater to tourists and locals. |
Improve operations | The CTA anticipates delays or surges in ridership. |
Reduce risks | Local Chicago banks assess the creditworthiness of residents to analyze potential loan defaults. |
Enhance decision-making | Real estate companies determine the potential value of properties in emerging neighborhoods, such as Woodlawn or East Garfield Park. |
Manage costs | Chicago hospitals, such as Rush, identify patients at high risk of readmission based on local health trends. |
You can have one or more objectives, but your priorities depend on the following:
- Urgent need
- Industry
- Resources available
- Changes within your organization
Despite artificial intelligence being an excellent marketing partner, the limitations and challenges of predictive analytics also dictate your goals. Some types of software might be incompatible with your data collection tools or impose limits on data size.
2. Collect the Data from Various Sources
The second step is gathering the data, which can be from internal or external sources. Internal sources are usually zero- and first-party information:
- Zero-party data means your leads and customers voluntarily provide it to you, perhaps when they signed up for the mailing list or completed a survey.
- First-party data are the ones you collect using different platforms, such as customer relationship management (CRM) systems and customer service interactions online and offline.
These are the least susceptible to legal risks of the different data types. They are also often reliable when measuring consumer behavior, interests, preferences, and needs.
Second- and third-party data are examples of external sources:
- Second-party data is the type of information derived from business partnerships. For example, a travel agency and a hotel can exchange demographic profiles.
- Third-party data usually comes from unrelated entities that have no direct relationship with the audience, business, or industry. These include lead-generation companies selling lists, governments, and news agencies.
Quality external data adds more context and insights, increasing the results’ accuracy.
How much data do you need to do predictive analytics? Usually, when we talk about this topic, we immediately think about big data. It is not always the case.
- Do you have time to clean and prepare the samples? If not, then big data is not for you.
- What do you value more: accuracy or information?
- How complex is your algorithm? What models do you plan to use?
- What problems are you trying to solve? If you want to predict sales within the next six months, historical performance from ten years ago is less likely to be useful.
3. Clean and Prepare the Data
With predictive analytics, it is garbage in, garbage out. You cannot expect it to give you relevant, accurate outcomes if the data is flawed to begin with.
Cleaning the datasets depends on the problems an analytics company in Chicago might find:
Data Problem | Solution |
---|---|
Missing values | Fill in the missing values using various techniques or replace them with the mode for categorical data. If they introduce bias, delete rows with missing information. |
Duplicates | Delete the duplicate. |
Outliers | Because they can skew the results, the agency might transform, remove, or try capping them. |
Inaccurate data | Correct or remove entries that are inconsistent with expected values. |
Issues with text data | Remove white spaces and special characters, correct typos, or standardize text. |
Preparing the information follows after cleaning. This one transforms the data into a format suitable for analysis. This could mean normalizing data scales, encoding categorical variables, or restructuring them to be compatible with the chosen analytical model.
AI platforms can clean and prepare information with ease. However, fresh eyes still matter in evaluating the value of the data for analysis, especially in terms of bias.
4. Pick the Best Predictive Modeling
Because predictive analytics often runs on machine learning, you can train it to provide more thorough, accurate, and timely forecasts over time. However, like any other training, it needs a guide, mentor, or teacher. In this case, it is your forecast model.
Here are some of the most popular options with their attributes, advantages, and disadvantages:
Forecast Model | Characteristic | Advantage | Disadvantage |
---|---|---|---|
Classic | Generates yes-and-no responses | Simple, modifiable, and easy to interpret | Prone to overfitting, where they perform exceptionally well on the training data but poorly on new data. |
Time-series | Predicts future values by analyzing observations at regular intervals over time | Effective for short-term predictions when data is consistent over time | Does not work well when data includes significant but irregular fluctuations |
Linear regression | Forecasts a dependent variable based on one or more independent ones | Provides insights into relationships between variables | Is sensitive to outliers |
Neural | Consists of layers of interconnected nodes, or “neurons,” that can learn patterns in data and sometimes present relationships visually | Models’ complex, non-linear relationships are effective for large datasets with high dimensionality | Requires extensive computational power and training time, and is less interpretable compared to simpler models |
With so many choices, how do you pick the best? Use these questions as your guide:
- What is your objective? Are you trying to understand the relationship between variables?
- Do you have outliers in your data?
- How much data do you have? Can it support a more complex model?
- Do you need real-time predictions, or is batch processing acceptable?
- Will the model need frequent retraining as new data becomes available?
- Do stakeholders or regulators need to understand how your models make decisions?
- How do you plan to validate the model?
5. Train, Evaluate, and Deploy the Model
Now that you have already exposed your model to data, you can move on to the last three steps.
First is training, which involves feeding the model with more information until it learns—that is, it displays more accurate, consistent results. During this stage, expect to continuously make corrections and set parameters. You can even push the system to determine how much information it can process until it begins to make mistakes.
After training, you assess its performance. Why? Sometimes the outcomes look good on paper, but they might not be what you expect or need. Doing this also determines your system’s ability to process unseen data.
An analytics company in Chicago usually evaluates the model in this manner:
- The team feeds the model the test set’s input data without revealing the outcomes.
- The model makes predictions based on its training.
- The team compares these predictions with the actual results to measure accuracy and precision, among other metrics.
These two processes can take weeks, months, or even years. It depends on many factors. Do the evaluation metrics meet your thresholds? For instance, in a medical diagnosis model, you might require high precision to minimize false positives. Can you scale the model?
If your answer to these questions is yes, it is ready for deployment. In other words, you can now integrate the system into your business infrastructure and marketing plan to start forecasting real-world data.
Summing Up
Predictive analytics makes you more competitive and even gives you the right tools to beat the leaders. It prepares you for potential significant market and economic changes and enhances the user experience.
These steps can get you started, but predictive analytics, as a whole, is challenging and complicated. For best results, work with an expert analytics company in Chicago. Contact Digital Authority Partners (DAP) for a free consultation.
Want To Meet Our Expert Team?
Book a meeting directly here
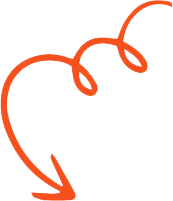