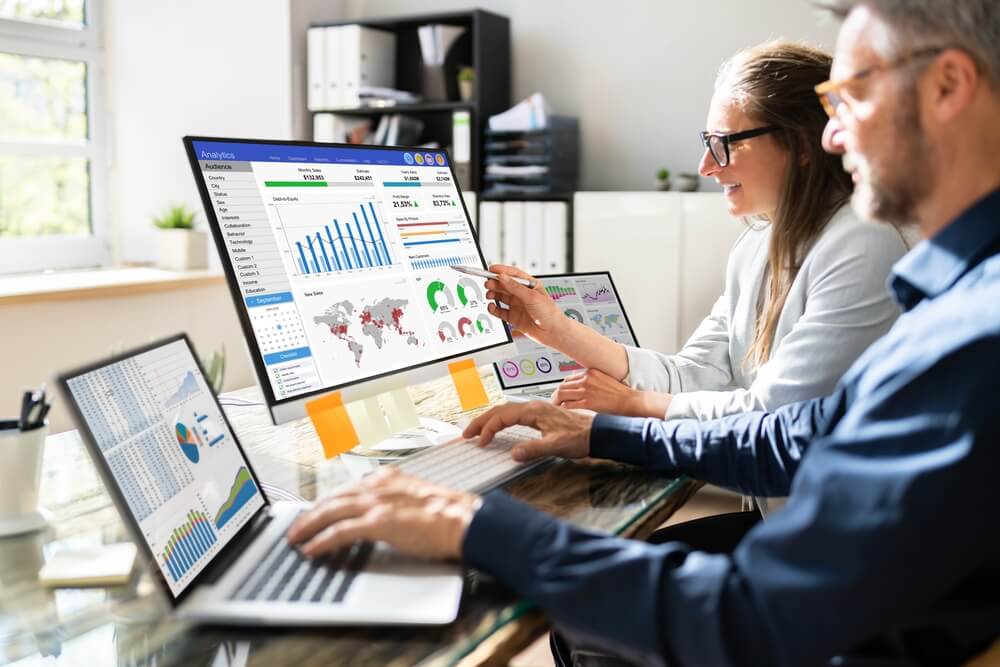
5 Practical Uses for Data Analytics, 5 Easy Analysis Methods
Data analysis gives businesses valuable insights into customer behavior, preferences, and market trends. Through data analysis, marketers can make informed decisions, create tailored marketing strategies to target specific customer segments, and optimize campaigns for better performance.
This article is about data analytics, highlighting the following topics:
Five practical uses of data analytics
- Customer segmentation
- Predictive analytics
- Churn analysis
- Website and user experience optimization
- Social media and sentiment analysis
Five easy data analysis methods
- Descriptive statistics
- Pareto analysis
- Data visualization
- Cohort analysis
- Regression analysis
Learn how an analytics company in Chicago can help you with data analytics. Let’s go!
5 Practical Uses for Data Analytics
Data analytics drive data-driven decision-making, which is critical in all businesses across industries. With effective data analytics, companies can achieve their goals and objectives.
1. Customer Segmentation
Data analytics help businesses identify customer segments according to user behavior, demographics, preferences, and buying patterns, helping companies tailor their marketing strategies. Here are some tips for performing customer segmentation using data analytics:
- Decide on the segmentation variables that are most relevant to the business goals.
- Gather relevant data for different segmentation variables. The data must be clean, complete, and accurate.
- Choose an appropriate segmentation method such as hierarchical clustering, k-means clustering, or decision tree analysis.
- Assess the homogeneity of each segment and validate distinctiveness by comparing the characteristics and behaviors of customers.
- Interpret findings and insights from the segmentation analysis to create tailored marketing strategies and campaigns.
2. Predictive Analytics for Sales and Demand Forecasting
Businesses can apply predictive analytics to forecast future sales and demands by analyzing historical sales data and market trends, optimizing the process, providing better customer service, and saving on costs.
Follow these strategies to use data analysis to perform predictive sales and demand forecasting analytics:
- Gather historical sales data and other relevant data related to sales.
- Check data for missing values, outliers, and inconsistencies.
- Create additional features or variables that enhance the model's predictive power.
- Select the appropriate predictive model for sales and demand forecasting.
- Split the data into training and validation sets.
- Monitor the model's performance and regularly update it with new data.
3. Churn Analysis and Customer Retention
Data analytics identifies customers at risk of leaving a business. Through customer behavior and engagement pattern analysis, companies are able to take proactive measures to retain customers.
Perform churn analysis and customer retention using data analytics by following these tips:
- Define what constitutes churn for the business, whether it's a canceled subscription, discontinued service, or non-renewal of a contract.
- Gather historical customer data, including sign-up dates, churn dates, customer interactions, and other relevant information.
- Segment the customer base determined by different attributes to identify high-risk groups and areas for improvement.
- Use data analysis techniques such as logistic regression or decision trees to identify the factors that drive churn.
- Develop targeted retention strategies based on the churn analysis's insights.
4. Website and User Experience Optimization
Data analytics offer insights into website traffic, user behavior, and interactions. Businesses can optimize their websites and user experiences with website and UX data.
Remember these strategies to make the most of data analytics for website optimization:
- Use website analytics tools such as Google Analytics to track user behavior on the website. Check page views, bounce rates, time on the page, and click-through rates.
- Conduct A/B testing to compare different versions of web pages, elements, or features.
- Analyze the conversion funnel, check for potential bottlenecks or drop-off points, and adjust to improve UX.
- Use heatmaps and session recordings to visually represent user interactions on the website.
- Gather feedback from website visitors through surveys, feedback forms, or user testing sessions.
5. Social Media and Sentiment Analysis
Data analytics monitors and analyzes social media conversations regarding a brand or product. Sentiment analysis gauges public opinion, identifies potential issues, or discovers missed opportunities.
Use these tactics to make the most of data analytics for social media and sentiment analysis:
- Monitor social media channels using tools to track mentions, comments, and interactions related to the brand across various social media platforms.
- Implement sentiment analysis algorithms to efficiently classify social media posts and comments into positive, negative, or neutral sentiments.
- Analyze trending topics and hashtags related to the brand or industry.
- Use segmentation on sentiment analysis results based on different user groups.
- Use sentiment analysis insights to promptly respond to all customer feedback.
5 Easy Analysis Methods
Various data analysis methods exist. The following are common in data analysis for marketing:
1. Descriptive Statistics
Descriptive statistics is a branch of data analysis that summarizes and describes the main features of a dataset, providing an overview of the data. Conduct descriptive statistics using these strategies:
- Decide which aspects of the data to describe such as numerical measurements (e.g., age, sales, income), or categorical attributes (e.g., gender, product categories).
- Calculate the measures of central tendency to understand the average value of the data.
- Analyze the spread of the data using measures such as range, variance, and standard deviation.
- Examine the data distribution through frequency charts to understand the data distribution across different values or categories.
- Use data visualization techniques to present descriptive statistics visually.
2. Pareto Analysis
Pareto analysis, or the 80/20 rule, is another popular data analysis technique to prioritize and focus efforts on the most significant factors. According to studies, roughly 80% of the effects come from 20% of the causes. Conduct Pareto analysis with these techniques:
- Define the issue you want to analyze such as product defects, customer complaints, and sales performance.
- Collect relevant data through customer feedback, sales data, defect reports, or any other relevant source.
- Quantify each factor's influence by calculating its frequency of occurrence or contribution to the problem.
- Create a Pareto bar chart that displays the factors in descending order of their influence to highlight the few vital factors contributing to most of the problem, enabling you to prioritize your efforts.
3. Data Visualization
This means creating a graphical representation of data and information to allow analysts and decision-makers to identify patterns, trends, and insights. Here are some tips to effectively perform data visualization:
- Select the most appropriate visualization type such as bar charts, line charts, pie charts, scatter plots, and heat maps.
- Keep visualizations simple and uncluttered.
- Use colors purposefully to emphasize key points and highlight patterns.
- Add meaningful titles, axis labels, and data annotations to provide context and make the visualization self-explanatory.
- Add interactive elements to allow viewers to explore the data further such as tooltips and filters.
4. Cohort Analysis
This groups data into segments, tracking the behavior of specific customer groups over time to understand trends and make targeted improvements. Here are ways to perform cohort analysis more effectively:
- Define cohort groups based on the criteria of time of acquisition, geographic location, product usage, or any other significant characteristics.
- Determine the critical metrics for each cohort, including customer retention, revenue per user, conversion rates, engagement levels, and more.
- Gather the relevant data for each cohort group and segment it accordingly.
- Normalize the data by dividing the metrics by cohort size.
- Present the cohort analysis results visually through charts or graphs.
5. Regression Analysis
This examines the relationship between a dependent variable and one or more independent variables. It determines how changes in one variable affect another and identifies factors that significantly influence the outcomes.
Follow these top tips to perform regression analysis:
- Define the hypothesis you want to investigate using regression analysis.
- Gather the relevant data for the variables of interest. Transform or preprocess the data as needed.
- Select the appropriate regression model such as linear regression, multiple regression, logistic regression (for binary outcomes), or polynomial regression (for nonlinear relationships).
- Evaluate the goodness of fit of the regression model to determine how well it represents the data.
- Interpret regression coefficients and their statistical significance. Determine the direction and strength of the relationship between the dependent and independent variables.
Summing Up
These practical uses and easy analysis methods show the overall value of data analytics in optimizing business operations, improving marketing campaigns, and enhancing customer experiences. With efficient data analysis, businesses can make informed decisions for sustained growth and success.
Partner with a trusted analytics company in Chicago for experienced data analysis. Contact Digital Authority Partners (DAP) today!
Want To Meet Our Expert Team?
Book a meeting directly here
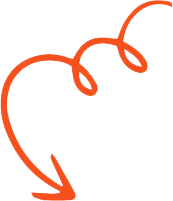