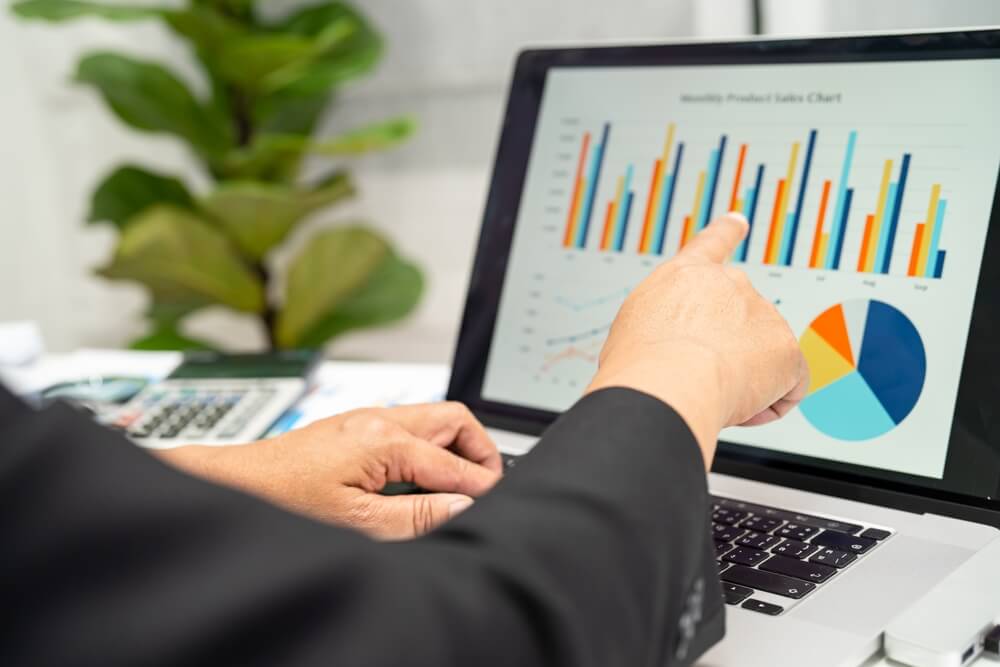
5 Basic Data Analysis Methods You Need for Greater Profits
Regardless of location and industry, a data-driven approach to business is a prerequisite to success. This idea rings truer for businesses in a highly competitive market such as Chicago. An analytics company in Chicago provides the expert guidance needed to harness the power of data analytics.
This guide delves into the five basic data analysis methods that have become integral to optimizing profits:
- Descriptive analysis.
- Diagnostic analysis.
- Predictive analysis.
- Prescriptive analysis.
- Exploratory data analysis.
- Practical steps in the implementation.
Let’s go!
1. Descriptive Analysis
Descriptive analysis provides a comprehensive summary of your company's historical data. It interprets long-term data trends and patterns to deliver insights into various business metrics.
One might view descriptive analysis as the foundation of data analysis. It interprets past data to describe what happened. This method of analysis is crucial for understanding the status quo of a business and the path it took to get there.
Descriptive analysis helps optimize profit by providing insights into operational efficiency, customer preferences, and market trends.
For example, a Chicago-based clothing retailer may use descriptive analysis to understand what products sold best in the past quarter. This information guides their inventory decisions for the next season.
Moreover, companies can leverage AI-driven data management systems for descriptive analysis. These systems work on vast amounts of data. They identify trends and provide reports with greater accuracy and speed.
2. Diagnostic Analysis
After understanding what happened, the next step is to figure out why it happened—a question answered by diagnostic analysis. This method digs deeper into the data to understand the cause of specific outcomes.
Diagnostic analysis involves more detailed data examination and advanced statistical techniques. Its primary purpose is identifying relationships and patterns among different variables to explain why a particular event occurred.
From a profitability perspective, diagnostic analysis allows businesses to pinpoint factors that positively or negatively affect their revenue.
For instance, a popular restaurant in Chicago might use diagnostic analysis to understand why sales spiked on certain weekends. Was it because of a particular event in town? Or did specific marketing efforts pay off?
Businesses that use data-driven marketing strategies are able to rely on diagnostic analysis to personalize marketing efforts. Doing so ensures the efficient use of resources and increases the likelihood of higher returns.
3. Predictive Analysis
Having understood what happened and why it happened, the next logical question to ask is, "What could happen?" This question brings us to predictive analysis. Predictive analysis involves using statistical models and forecasting techniques to understand the future.
This analysis method allows businesses to forecast future outcomes based on historical data and trends. This predictive capability is invaluable, especially when it comes to financial planning, risk management, and strategic decision-making.
Predictive analysis plays a vital role in profit maximization. For instance, a Chicago-based logistics company uses this method to forecast fuel prices. With this information, they can plan their operations to maximize profit margins during periods with lower predicted fuel costs.
Similarly, a retail store has the ability to forecast future sales trends based on historical data, enabling them to manage inventory more efficiently. This not only reduces costs but also ensures that the right products are available when customers want them.
4. Prescriptive Analysis
The final question to ask is, "What should we do?" Prescriptive analysis provides the answer. As the pinnacle of business data analysis, it enables decision-makers to anticipate what will happen and when. Most importantly, it helps them understand why it will happen.
Prescriptive analysis goes beyond predicting future outcomes. It uses sophisticated techniques to suggest actions based on predictions and potential outcomes. Some of these techniques include machine learning (ML) and algorithms.
This data analysis method optimizes profits by providing businesses with a roadmap of the best possible actions based on their data. A Chicago-based ecommerce platform, for example, may use this method to determine the best pricing strategy for a festive season sale, ensuring maximum profitability.
Equally, a pharmaceutical company might use prescriptive analysis to optimize its supply chain. Doing so ensures the timely delivery of products to distributors and reduces storage and transportation costs. The power of prescriptive analysis lies in its ability to turn data-driven insights into actionable, profit-boosting strategies.
5. Exploratory Data Analysis
Exploratory Data Analysis (EDA) is essential to understanding the various aspects and patterns within an extensive data set. Think of this method as a preliminary investigation, where one sifts through data to discern patterns, identify anomalies, or verify assumptions.
For profitability, EDA reveals business trends and customer behavior patterns that might not immediately be apparent.
Take, for example, a Chicago purveyor of artisanal soaps that sees a fluctuating sales pattern over the year. With the application of EDA, this enterprise can investigate patterns in more detail. It might find that certain fragrances perform better during specific seasons.
Armed with this information, the enterprise may wisely choose to modify its production schedules to align with seasonal demand for different scents. Such adjustments can enhance sales performance, translating into augmented profit margins.
Practical Steps to Implement These Data Analysis Methods
Integrating data analysis methods into your daily business operations may seem daunting. However, with the following step-by-step guide, you can systematically implement these methods:
1. Understand Your Objectives: Clearly define the business problem you aim to solve with data analysis. Your objectives could range from reducing operating costs to increasing profit margins.
2. Gather Quality Data: Implement robust data collection and management systems to gather the necessary data for your analysis. Make sure that your data is accurate and relevant to your objectives.
3. Choose the Appropriate Data Analysis Method: Select the method that best suits the nature of your problem and the type of data you have. For instance, use diagnostic analysis to understand why sales are declining.
4. Use Suitable Tools and Software: Employ platforms such as Tableau, Microsoft Power BI, and Google Analytics. These tools offer sophisticated yet user-friendly data analysis features.
5. Address Potential Challenges: Implementation might present challenges such as data privacy concerns or skill gaps within the team. Proactively address these challenges by investing in data privacy technologies, providing staff training, or even bringing in third-party experts when needed.
Summing Up
Embracing these basic data analysis methods can lead to significant profit enhancements. Businesses have to adapt to data-driven decision-making for sustainable growth. If you require assistance implementing these strategies, do not hesitate to contact Digital Authority Partner (DAP). Let us discuss how our experts can improve your profitability through data analysis.
Want To Meet Our Expert Team?
Book a meeting directly here
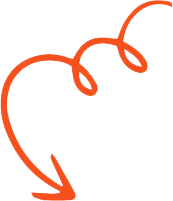