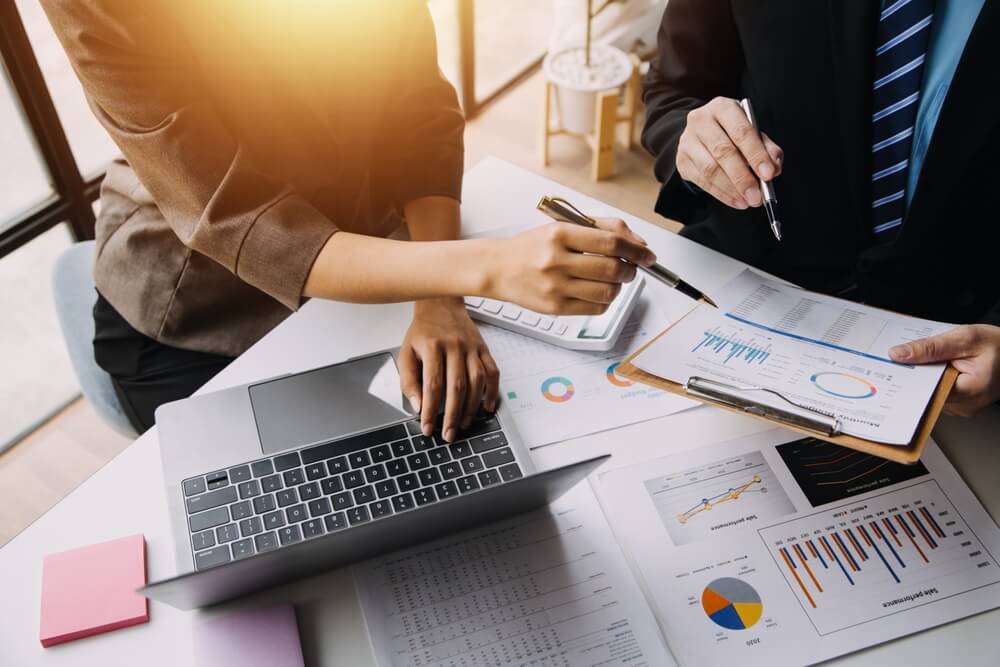
How Companies Successfully Use Analytics To Predict Sales
Businesses need accurate sales predictions to succeed in today's highly digitalized markets. The Chicago market is one such market. Navigating the city’s data-driven landscape requires companies to effectively use data analytics. With the help of an analytics company in Chicago, businesses gain insights into future sales performance.
This guide explores how companies use data analytics to reliably predict sales. It talks about the following:
- Defining objectives
- Gathering relevant data
- Applying analytics techniques
- Testing and validating models
- Integrating into decision-making
- Collaboration across departments
- Agility and adaptability
- Continuous improvement
Let’s go!
Are you looking for a full-service digital marketing agency? See the DAP difference below!
Defining Objectives for Sales Prediction
In a marketplace as competitive as Chicago, businesses must clearly define their goals and metrics for sales prediction. The initial step is aligning sales prediction objectives with the overall business strategy, which can include data-driven marketing efforts.
For example, a Chicago restaurant aiming to increase its takeout sales might set objectives for predicting weekly or monthly takeout sales volumes. This alignment ensures that every piece of data gathered and analyzed is purposeful and propels the company toward its primary goals.
Gathering and Preparing Relevant Data
Successful sales prediction hinges on the quality of the data at a company's disposal. The type and volume of relevant data can vary greatly in the Windy City, where businesses range from tech startups to established manufacturing firms. Companies must identify and collect data pertinent to their business model and industry.
For instance, a retailer on the Magnificent Mile might find value in historical sales data, customer demographic information, and seasonal shopping trends. Ensuring the accuracy, consistency, and completeness of this data is crucial. Businesses then must use data cleaning and preparation techniques to create a reliable foundation for the impending analysis.
Implementing Analytics Techniques
Predicting sales involves using different analytical methods. These methods should fit the business type and its sales system, especially in a varied business environment such as Chicago's.
One method, statistical analysis, helps study past sales data to spot noticeable trends. Take, for example, a bookstore that notices a regular sales increase during a citywide annual literary event.
Another method, regression modeling, helps businesses understand the link between sales and various factors that can affect them. For instance, a car dealership can use this method to see how changes in interest rates or fuel prices have affected its past sales.
Moreover, businesses use time-series analysis to predict future sales on the basis of past trends. Businesses that observe distinct seasonal sales patterns frequently use this technique.
Finally, machine learning algorithms help companies create predictive models by considering many variables. A technology company, for example, can predict its software sales by looking at a range of factors such as market demand, competitor pricing, and its own marketing spending.
Testing and Validating Predictive Models
After creating predictive models, testing and validating them are important. These two usually involve splitting the collected data into two parts: one for training and one for testing. The training set underpins model building, while the testing set checks model performance.
Companies then compare the sales predictions made by the model with the actual sales data. Take the example of a brewery that predicts its craft beer sales using regression modeling. By comparing the sales the model predicted with the actual sales, the brewery can assess how accurate the model is.
However, the work does not stop here. Businesses might need to tweak and fine-tune predictive models based on how well they perform to improve their accuracy. Regular checks ensure that these models remain reliable for predicting sales.
With accurate models, businesses across the Chicago area can confidently predict their sales, improving their strategic planning and decision-making.
Integrating Predictions into Decision-Making Processes
Once a predictive model is accurate and reliable, companies can confidently integrate these insights into their strategic planning. Businesses in Chicago and beyond have found tremendous value in aligning their strategies with predictive data, particularly in key areas such as sales, marketing, and finance.
Sales predictions can shape a company's future direction. For example, predictions of increased sales in the upcoming quarter might justify additional investments in production or staffing. On the other hand, a forecasted downturn might prompt the company to cut costs or explore new revenue streams.
Collaboration and Cross-Departmental Alignment
Successful sales prediction is not solely the responsibility of the analytics team. Rather, it requires cooperation and alignment across all departments. In particular, sales, marketing, finance, and analytics must work together. When these teams collaborate and share insights, companies can create a comprehensive sales forecast considering many factors.
Take a Chicago-based retail company, for instance.
- The sales team provides on-the-ground insights about customer preferences and buying behavior.
- The marketing team has data on campaigns and customer engagement.
- The finance team understands the financial implications of sales trends.
- The analytics team synthesizes all these insights into a coherent, data-driven prediction.
Agility and Adaptability in Sales Prediction
Like in any major city, the business landscape in Chicago is dynamic and subject to change. Thus, successful companies keenly monitor evolving market trends and customer behaviors. They are prepared to update their predictive models in response to new data and changing circumstances.
This agility is essential to staying competitive. Suppose a Chicago-based food delivery service sees a significant increase in orders due to a change in weather patterns. This business can adjust its sales predictions and operations to meet the increased demand and maximize profits.
Continuous Learning and Improvement
With each sales cycle, companies gain more data and the opportunity to refine their predictive models. The most successful companies adopt a mindset of continuous learning and improvement. They recognize that each prediction and its outcome provide valuable insights into whether the prediction was accurate.
For instance, a Chicago-based software company has predicted slower sales during a certain period. If sales exceeded expectations, the company would examine what factors contributed to this growth. These factors can include a successful marketing campaign or increased demand for digital solutions. These findings inform sales forecasts.
This way, analytics and sales predictions become cyclical processes. Each cycle offers opportunities to refine and improve the predictive models and strategies. Companies that embrace this process position themselves to be more responsive, strategic, and successful in their sales efforts.
Summing Up
Using analytics for sales prediction gives any business an edge. It delivers businesses crucial insights, helping them predict sales with great precision. The process fine-tunes business plans.
A data-driven approach to sales forecasts is essential in today's competitive market. If your business is ready to invest in analytics, contact Digital Authority Partners today. As a leading analytics company in Chicago, we are prepared to help you make smart decisions that push your business forward.
Want To Meet Our Expert Team?
Book a meeting directly here
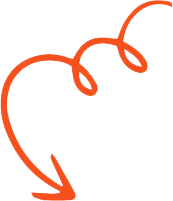